NCCS Collaborates with the
NSF Spatiotemporal Innovation Center
at George Mason University
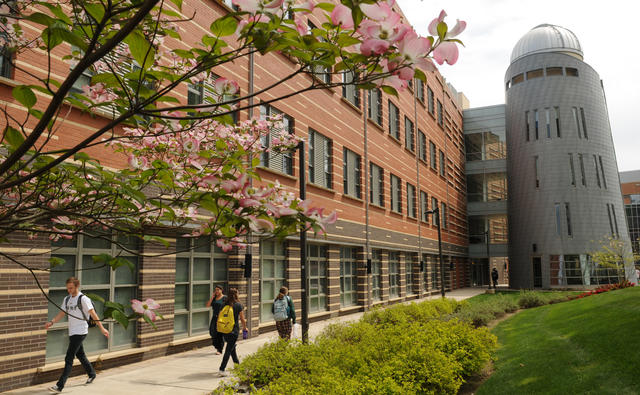
Research Hall on the Fairfax, Virginia campus of George Mason University (GMU) hosts the NSF Spatiotemporal Innovation Center. Photo courtesy of the GMU College of Science.
Summary
The NASA Center for Climate Simulation (NCCS) is a member and sponsor of the NSF Spatiotemporal Innovation Center (STC) at George Mason University (GMU), a collaborative research center spearheaded by Director Phil Yang. This seven-year NCCS/STC partnership has resulted in collaboration on many innovative machine learning (ML) research projects that include the Climate Data Downscaling Project, featured here, and the Cloud Classification Project, which will be described in a future highlight. The STC and its members are also providing support for the COVID-19-related Spatiotemporal Rapid Response Gateway.
Background
Under the National Science Foundation (NSF) Industry-University Cooperative Research Center (I/UCRC) program, George Mason University is one of two centers within the STC, with the other site located at Harvard University. NSF provided support and seed funding for the STC, and through the STC mechanism, Phil Yang founded the GMU Center for Intelligent Spatial Computing.
Yang has formed official partnerships between the STC at GMU and its member organizations including the NCCS, other governmental entities, and private sector companies such as RMDS Lab. The NCCS and other STC members provide funds, data, computational resources, ideas, and expertise to support a variety of collaborative research projects. One such effort, the Climate Data Downscaling Project, promises better, more accurate local precipitation data modeling and forecasting through a new machine learning method.
Climate Data Downscaling Project
Since most climatic precipitation products are too coarse for local hydrological impact studies, they require “downscaling” to obtain higher resolutions. Traditional precipitation downscaling methods such as statistical and dynamic downscaling require an input of additional meteorological variables, and very few are applicable for downscaling hourly precipitation for higher spatial resolution.
NCCS staff worked with STC scientists on this collaborative effort in 2018–2019, with the goal of experimenting with and ultimately developing a better method of downscaling hourly precipitation data for regional climate impact studies. The project used existing “reanalyzed” data—a synthesized, comprehensive estimate of the state of the Earth system based on observations and a numerical model. The reanalyzed data was derived from global climate models (GCMs) with 10-kilometer spatial resolution and containing long-term historical climate records.
Process
The Climate Data Downscaling Project began with investigating and utilizing a global reanalysis dataset, the Modern-Era Retrospective Analysis for Research and Applications, Version 2 (MERRA-2), generated by the Global Modeling and Assimilation Office (GMAO) and supplied by the NCCS. GMAO and NCCS experts helped STC researchers understand and use this long-term, historical, simulated meteorological dataset.
For this project, a team of researchers from the GMU STC, alongside GMAO scientists and NCCS staff, developed a new ML-based downscaling method, PreciPatch. The team tested and validated PreciPatch using MERRA-2 data and compared its performance with two other downscaling methods: RainFARM—a stochastic downscaling method; and DeepSD—a Super-Resolution Convolutional Neural Network (SRCNN)-based downscaling method.
The team used four years of GCM precipitation data (2014–2017) recorded at an hourly temporal resolution over four different geographic areas as the training dataset and conducted the initial processing of precipitation downscaling using the NCCS Advanced Data Analytics Platform (ADAPT). PreciPatch is based on dynamic dictionary learning, and researchers adopted this machine learning (ML) method to build a dictionary of the relationships between low- and high-resolution subsets—or patches—of the original GCM datasets. By assimilating more datasets, the dictionary was enhanced and its prediction accuracy was increased, in terms of local precipitation modeling. The enhanced dictionary was then used to downscale low-resolution GCM data for a defined time interval and produce results.
Results

A visual comparison of various methods used to downscale regional global climate model data to local precipitation clusters at various scales. Graphic from Mengchao Xu, Spatiotemporal Innovation Center, George Mason University.
Scientists collaborating through the STC found that the PreciPatch method demonstrated better performance than RainFARM and DeepSD for downscaling short-duration precipitation events, as illustrated in the graphic above and the table below.
Bicubic | DeepSD | RainFARM | PreciPatch | |
---|---|---|---|---|
Training Time | N/A | ~ 1 hour for 3 months of data (national coverage) | N/A | ~200 hours for 300 million patches (national coverage) |
Applicability to Different Areas | Directly apply, no training needed | Additional training needed if the area is different or input size is different | Directly apply, no training needed | Directly apply, no need for additional training |
Computing Time | < 0.001 sec per grid point | ~ 0.005 sec per grid point | ~ 0.005 sec per grid point | ~ 2 sec per grid point per process |
Overall Downscaling Performance | Baseline | Not good—close to the baseline | Rainfall clusters are not well-simulated | Best |
Holds Rainfall Consistently with Large-scale Input? | No | No | Yes | Yes |
A statistical comparison between various methods used to downscale regional GCM data to local precipitation clusters at various scales. Table from Mengchao Xu, Spatiotemporal Innovation Center, George Mason University.
The Future
This collaborative project between NCCS staff and STC researchers will help climate scientists use GCMs and ML-based downscaling methods to study, model, and forecast local precipitation impact on the environment in a changing climate, which is key to managing agricultural and water resources. The various advantages of PreciPatch as a downscaling method include implementing the process on actual data; constructing a single, master dictionary that can be further enriched; and eliminating the need for additional climate variables or probability assumptions in the downscaling process. The ML-based data dictionary developed through this project can be further enriched and optimized; thereby better and more accurate local precipitation data can be modeled and forecasted using PreciPatch.
“PreciPatch data downscaling helps address the increasing demand for short-term weather forecasts with high spatial and temporal resolutions,” STC Director Phil Yang observed. “The key, I think, is that the research results from the NCCS/STC Climate Data Downscaling Project can be used to help stand up a gateway with relevant data analytics, ML results, information, and knowledge produced specifically for climate scientists.”
Spatiotemporal Rapid Response Gateway
Currently, the STC is also working with Harvard University and a cadre of global members to share data and computing resources through the COVID-19 Spatiotemporal Rapid Response Gateway—helping to collect, integrate, analyze, and provide critical and unique COVID data to the global community to address and understand the epidemic. As an STC member, the NCCS is helping to partially fund this project.
Related Link
Mengchao Xu, Q. Liu, D. Sha, M. Yu, D. Duffy, W. Putman, T. Lee, and C. Yang: PreciPatch: A Dictionary-based Precipitation Downscaling Method. Remote Sens., 12, e2020/03/23, doi: 10.3390/rs12061030.
Sean Keefe, NASA Goddard Space Flight Center